Poor Data Quality Can Have Long-Term Effects
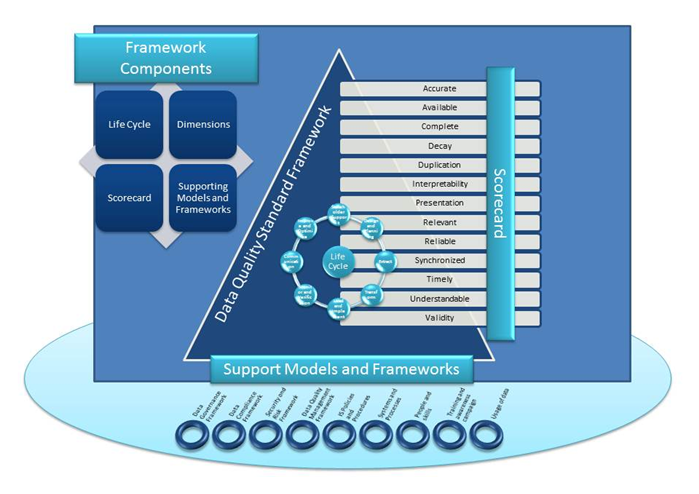
Ever-changing customer data
As much as you hear about the importance of data quality being the determinant of your organization’s success, companies all over the world still use inaccurate and outdated data in their daily work. In the bulk of information that piles up over the months, even years, you usually can’t even identify what’s more urgent and important.
The traditional ETL approach, which quite a few companies have come to use, sure is helpful. But once you’ve gone through its stages, it’s important not to forget that your new cleansed data is still constantly being enriched and changed. So, in less than no time, a new challenge emerges as you get your cleansed data mixed with new data that isn’t necessarity as consistent and reliable as it should be.
At the same time, Gartner estimates that 50% of enterprises undertaking a CRM strategy are unaware of significant data quality problems in their systems. According to Bernie Gracy of Pitney Bowes Group, these consequences may influence C-level executives of various roles. “The effects of poor data can have long-term, devastating consequences,” Bernie says.
“Recipients of duplicate mailings are likely to become frustrated and question the firm’s overall operating efficiency. If these redundant mailings each consistently misspell the individual’s name or address, the frustration level is likely to approach alienation or even a legal concern—especially if the recipient had previously made a request to the mailer that they be removed from the vendor’s mailing list or asked to be placed on an industry-wide, do-not-mail list.” —Bernie Gracy, Pitney Bowes Group
Many of these inefficiencies may lead to the loss of credibility, significant fines, and even lawsuits.
“Data inefficiencies can also result in missed up-sell and cross-sell opportunities. Without a single view of the customer across the enterprise, it’s impossible to aggregate information to make decisions. This makes it impossible to distinguish between single-product and multi-product buyers, or between new and existing customers.” —Bernie Gracy, Pitney Bowes Group
How to address this
What should you do? Analyzing many expert research studies and customer involvements, we see that implementing a unified and repeated data quality monitoring approach is important. Here’s a great schema found on Wikipedia, confirming the need for an integrated approach.
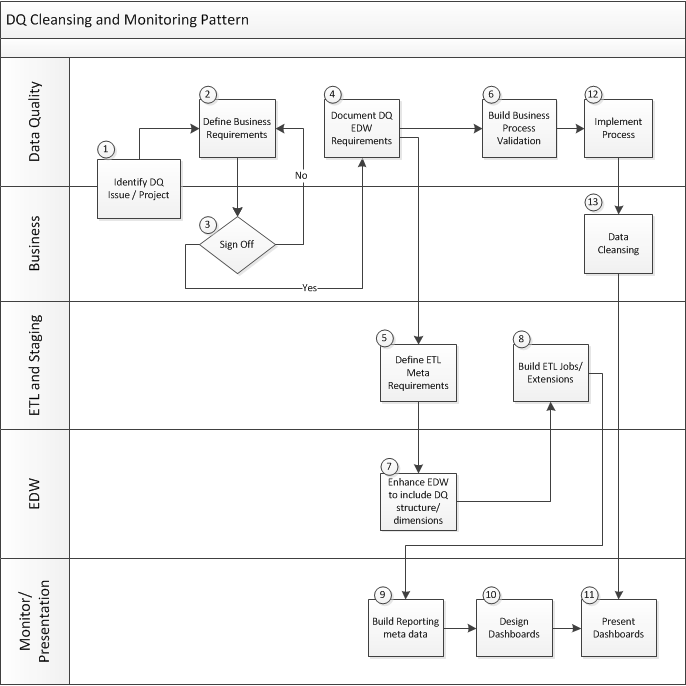
The steps you could follow while at that include:
- Create a clear standard that your incoming data should match
- Identify the main issues with incoming data by checking it against the created standard
- Look for the ways to solve the identified problems (as a possibility you cold create a notification system to send out alerts whenever unvalid or inconsistent data is detected)
At the first glance, this looks like it could solve your problems. But that’s just your incoming data. Another part of the problem lies in the clean data already stored in your warehouse. It’s validity isn’t everlasting, is it? Thus, a few more things in your to-do list:
- Identify the most appropriate time span for your data to be re-verified
- Schedule your data verification system to conduct repeated checks according to the identified data validity time span
All in all, just keep in mind that data within any organization is a dynamic and constanly changing asset, and data quality checking should become a repeated procedure, rather than a one-time practice.
Think about it in advance
Has it ever crossed your mind that the quality of data needs to be thought through before it’s actually gathered? That’s new and you don’t see too many companies thinking of it…yet. We don’t quite realize that even at the early stages of preparing for data gathering and while obtaining it, data quality aleady plays an important role in the future of your company. It is the early stages that make a difference in what your data become in the end. If approached properly from the very beginning, your data will surely pay off when you get to sharing and maintaining it, and especially when applying it.
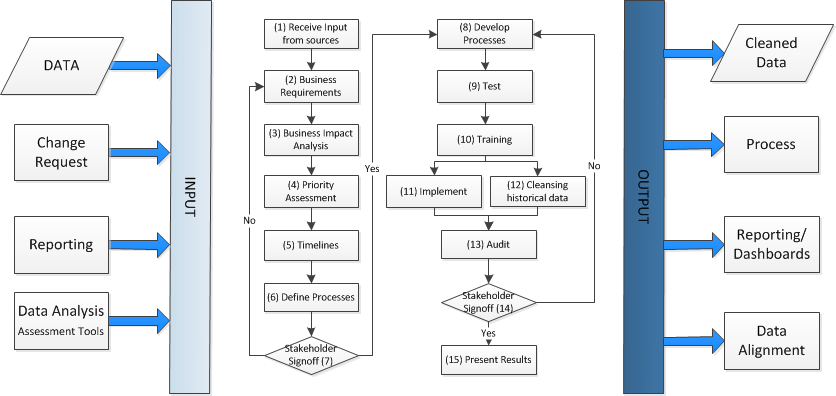
Cost-wise, this approach is rather efficient, too. Corporate data is not the thing to save up on—investing in data quality from the very beginning would save up a lot when it comes to verification, cleansing, and use. Cleansing, as a matter of fact, may very well become redundant. Sweet?
Further reading
- Neglecting the Quality of Data Leads to Failed CRM Projects
- Data Quality: Upstream or Downstream?
- 5 Things to Watch Out for in Data Warehousing