The Reasons for Poor Data Quality
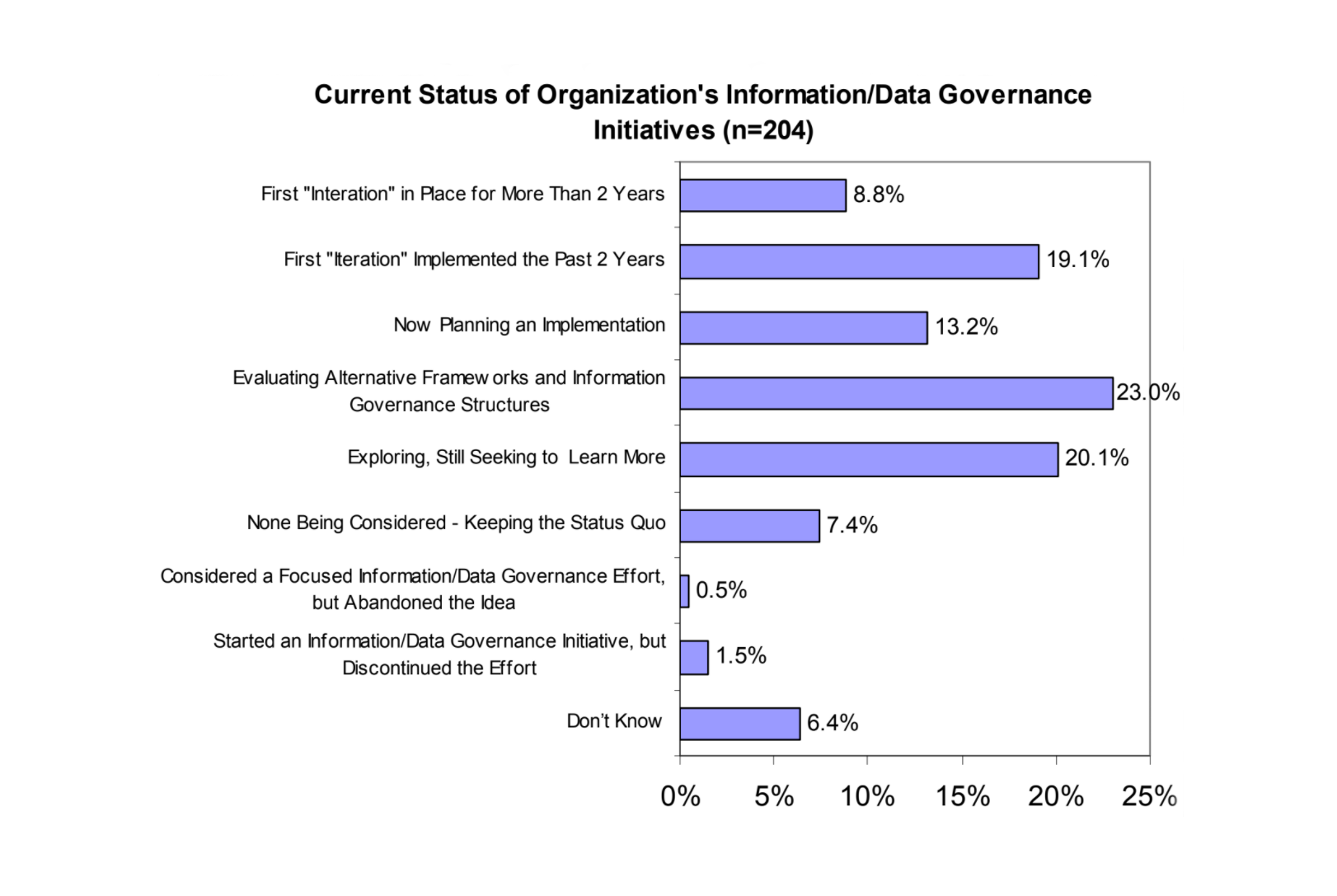
Data quality is still an issue
Lately, there have been talks about the need for intersection between data integration and data quality functionality. True, no integration initiative can be called successful without the accuracy and relevance of the information provided. The benefits of this consolidation are quite obvious: a consistent and complete view of corporate data, and, as a result, the ability to improve business processes faster to conquer the markets.
Some steps have already been made toward binding technology offerings and enhancing their features, however, data management still remains a challenge today. According to Gartner, three-quarters of F1000 companies struggle with data quality, having 25% of their critical data inaccurate, duplicated, or incomplete. Last year’s report from the International Association for Information and Data Quality revealed that data governance initiatives were still at their early stages.
The reason lies in the difficulty of understanding what quality data is and in estimating the cost of bad data. It isn’t always clear why or how to correct this problem, because poor data quality presents itself in many different ways.
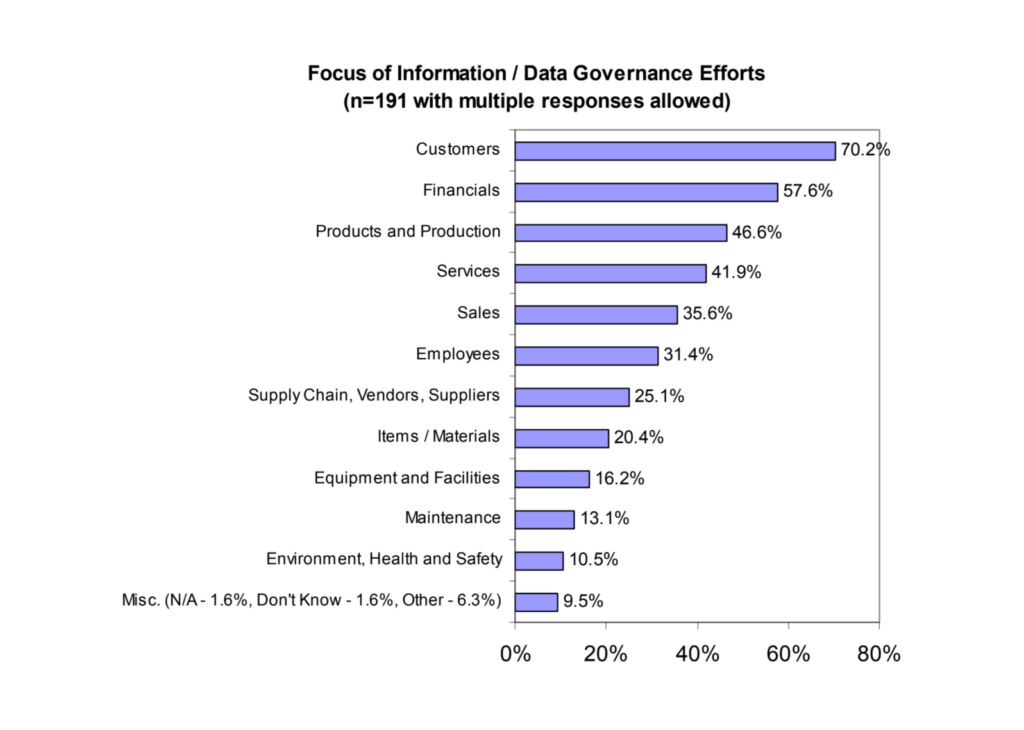
A better understanding of the underlying sources that generate quality issues can help develop a proactive and strategic plan to address the problem.
Seven sources of poor data
Last month, I stumbled upon an article by William McKnight, where he describes the seven possible sources of poor data quality. Here they are, in the order of their occurrence:
- Entry quality—usually caused by a person entering data into a system. The problem may occur due to a typo or a intentional decision, such as providing a dummy phone number or address. Identifying these outliers or missing data is easily accomplished with profiling tools or simple queries.
- Process quality—such issues occur systematically as data moves through an organization. They may result from a system crash, file loss, or any other technical accident that results from integrated systems. These problems are often difficult to identify, especially if the data has made a number of transformations on the way to its destination. Process quality can usually be remedied easily once the source of the problem is identified. Proper checks and quality control at each touch point along the path is needed to ensure that problems are rooted out, though these checks may often be absent in legacy processes.
- Identification quality—resulting from a failure to recognize the relationship between two objects. For example, two similar products with different SKUs are incorrectly judged to be the same. Identification quality may have significant associated costs, such as mailing the same household more than once (learn how to address this here). Data quality processes can largely eliminate this problem by matching records, identifying duplicates, and placing a confidence score on the similarity of records.
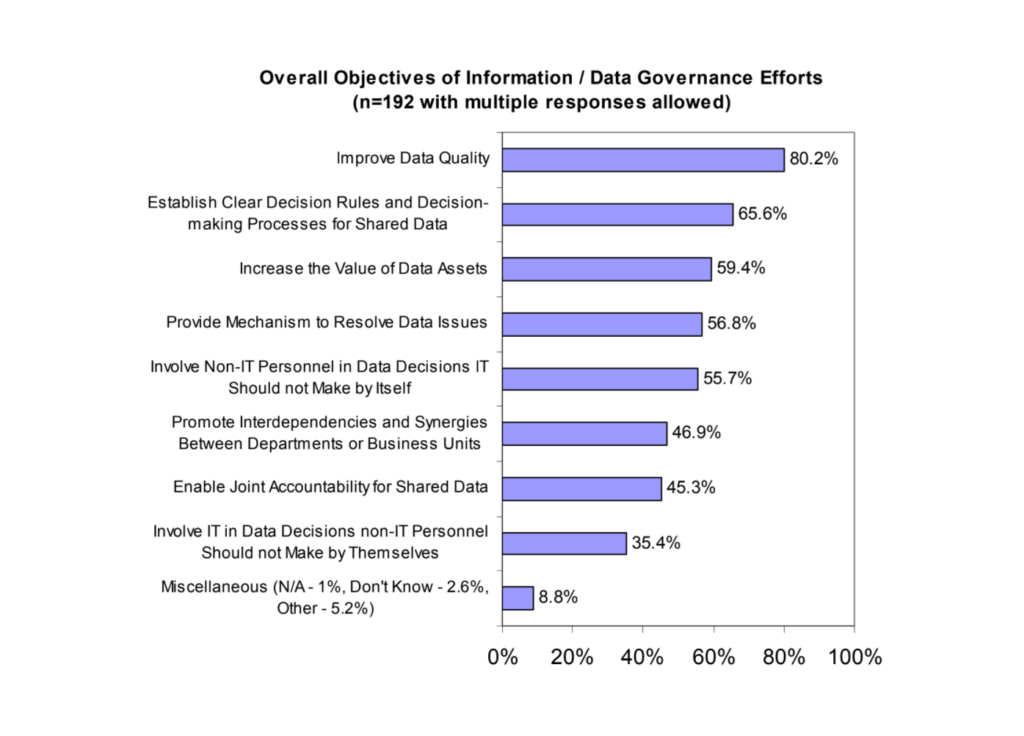
- Integration quality—occurring due to failed integration of all the known information about an object, which, normally, should be done to provide an accurate representation of the object.
- Usage quality—caused by incorrect use and interpretation of the information at the point of access.
- Aging quality—occurring as the information that can no longer be trusted due to the fact that time passes and the information become outdated.
- Organizational quality—which may occur when the information is reconciled between two systems based on the way the organization constructs and views the data.
Today, data quality is no longer a domain of just a data warehouse or ETL—it is accepted as an enterprise-wide responsibility. Having necessary tools, experience, and best practices, companies can address the issues with ‘dirty’ data before it reaches decision-makers.
Further reading
- Data Quality: Upstream or Downstream?
- Solving the Problems Associated with “Dirty” Data
- MDM Longs for Data Quality and Solid Grounds