Learning Game Control Strategies with Deep Q-Networks and TensorFlow
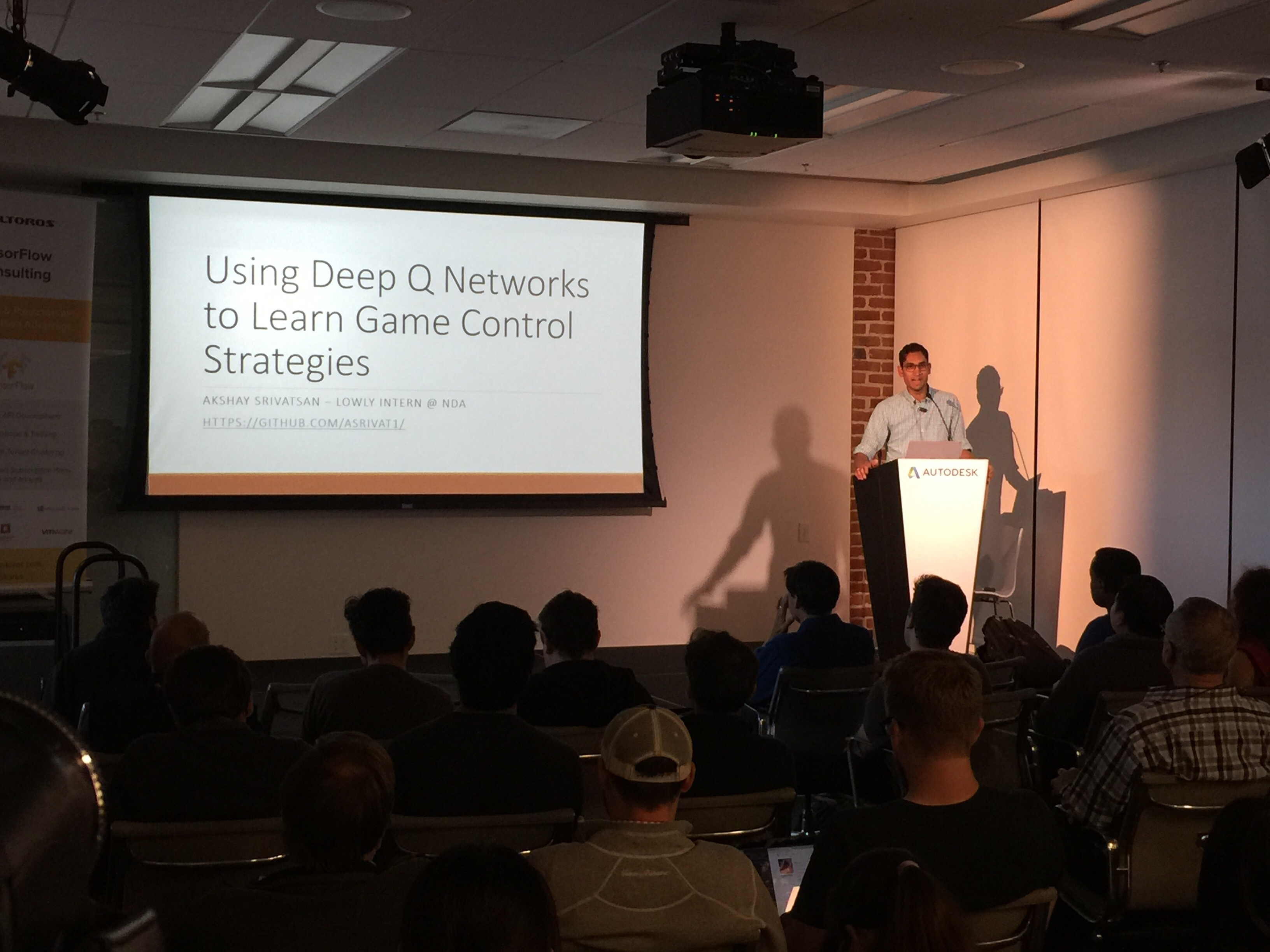
Reinforcement learning is an area of machine learning that enables an agent to learn how to interact with its environment—based on positive and negative feedback from the interactions. A recent TensorFlow San Francisco meetup was dedicated to deep Q-learning as a reinforcement learning technique and to the implementation of this model using TensorFlow.
Q-learning for video game environments
In his presentation, Akshay Srivatsan focused on reinforcement learning. He also talked about a recently developed deep learning model to find out optimal control patterns from visual input—using reinforcement learning and, precisely, deep Q-learning. According to Akshay, this technique is highly generalizable and is capable of achieving better than human performance in several specific video game environments.
In addition, Akshay showed how the discussed model can be implemented using the TensorFlow library. For the details on employing deep Q-networks to learn video game strategies and on the achieved results, see this GitHub link.
Among other topics on the meeting agenda were also the latest advancements in reinforcement learning, such as Google’s AlphaGo, which beat Lee Sedol—the 18-time Go world champion—in March, 2016.
Akshay’s presentation slides are also available.
Want details? Watch the video!
Further reading
- What Is Behind Deep Reinforcement Learning and Transfer Learning with TensorFlow?
- Mastering Game Development with Deep Reinforcement Learning and GPUs
- Deep Q-Networks and Practical Reinforcement Learning with TensorFlow
- TensorFlow in Action: TensorBoard, Training a Model, and Deep Q-learning
Related slides
About the expert